
The integration and application of large language models (LLMs) in medicine and healthcare has been a topic of great interest and development.
As noted at the Healthcare Information Management and Systems Society World Congress and other notable events, companies like Google are leaders in exploring the potential of generative AI in healthcare. Their initiatives, such as Med-PaLM 2, highlight the evolving landscape of AI-driven healthcare solutions, particularly in areas such as diagnostics, patient care, and administrative efficiency.
Google’s Med-PaLM 2, a leading MBA program in healthcare, has demonstrated impressive capabilities, particularly achieving “expert” level on questions modeled on US medical licensing exams. This model and others like it promise to revolutionize the way health care professionals access and use information, potentially enhancing the accuracy of diagnosis and the efficiency of patient care.
However, alongside these developments, concerns have been raised about the practicality and safety of these techniques in clinical settings. For example, relying on extensive Internet data sources for model training, while useful in some contexts, may not always be appropriate or reliable for clinical purposes. As Nigam Shah, MD, MBBS, senior data scientist at Stanford Healthcare, points out, critical questions to ask relate to the performance of these models in real-world medical settings and their actual impact on patient care and healthcare efficiency.
Dr. Shah’s perspective underscores the need for a more personalized approach to the use of LLM. Instead of general-purpose models trained on broad Internet data, he proposes a more focused strategy where models are trained on specific, relevant medical data. This approach is similar to training medical trainees – providing them with specific tasks, supervising their performance, and gradually allowing them more independence as they demonstrate competence.
In line with this, the development of Meditron by researchers at EPFL represents an interesting advance in this field. Meditron, an open source LLM software designed specifically for medical applications, represents an important step forward. Trained on curated medical data from reputable sources such as PubMed and clinical guidelines, Meditron offers a more focused and potentially more reliable tool for medical practitioners. Its open source nature not only promotes transparency and collaboration, but also allows for continuous improvement and stress testing by the broader research community.
MEDITRON-70B achieves an accuracy of 70.2 on USMLE-style questions in the MedQA-4-options dataset.
The development of tools such as Meditron, Med-PaLM 2 and others reflects a growing recognition of the unique requirements of the healthcare sector when it comes to AI applications. The focus on training these models on relevant, high-quality clinical data, and ensuring their safety and reliability in clinical settings, is crucial.
Furthermore, the inclusion of diverse datasets, such as those drawn from humanitarian contexts such as the International Committee of the Red Cross, shows sensitivity to the diverse needs and challenges in global healthcare. This approach is consistent with the broader mission of many AI research centers, which aim to create AI tools that are not only technologically advanced but also socially responsible and useful.
The paper, “Large Language Models Encoding Clinical Knowledge,” recently published in Nature, explores how large language models (LLMs) can be used effectively in clinical settings. The research provides pioneering insights and methodologies, and highlights the capabilities and limitations of LLM in the medical field.
The medical field is characterized by its complexity, with a wide range of symptoms, diseases and treatments that are constantly evolving. LLM degree holders must not only understand this complexity, but also keep up with the latest medical knowledge and guidelines.
The core of this research revolves around a newly harmonized standard called MultiMedQA. This standard combines six existing datasets for answering medical questions with a new dataset, HealthSearchQA, which includes medical questions that are frequently searched online. This comprehensive approach aims to evaluate LLMs across different dimensions, including realism, understanding, reasoning, potential harm, and bias, thus addressing the limitations of previous automated assessments that relied on limited criteria.
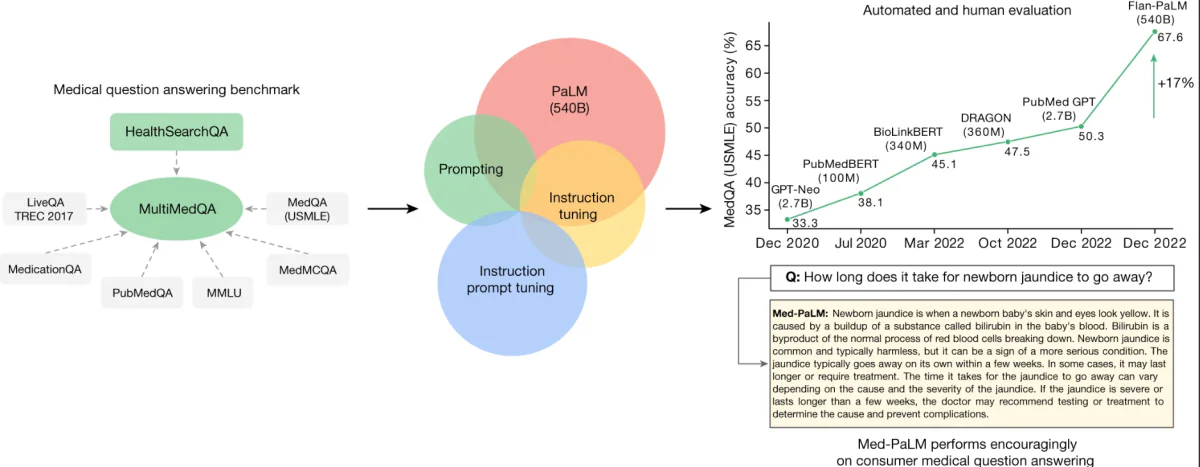
MultiMedQA, a standard for answering medical questions that includes medical examination
Key to the study is the evaluation of the Pathways Language Model (PaLM), a 540-billion-parameter LLM, and its instruction-tuned variant, Flan-PaLM, on MultiMedQA. Remarkably, Flan-PaLM achieves state-of-the-art accuracy on all multiple-choice datasets within MultiMedQA, including 67.6% accuracy on MedQA, which includes questions modeled on US medical licensing examinations. This performance represents a significant improvement over previous models, exceeding the previous state of the art by more than 17%.
MedQA
Format: question and answer (Q + A), multiple choice, open domain.
Example question: A 65-year-old man with hypertension comes to the physician for a routine health maintenance examination. Current medications include atenolol, lisinopril, and atorvastatin. His pulse is 86 min−1, respirations are 18 min−1, and blood pressure is 145/95 mmHg. Cardiac examination reveals end diastolic murmur. Which of the following is the most likely cause of this physical examination?
Answers (correct answer in bold): (A) Decreased compliance of the left ventricle, (B) Myxomatous degeneration of the mitral valve (C) Inflammation of the pericardium (D) Dilation of the aortic root (E) Thickening of the mitral valve leaflets.
The study also identifies critical gaps in the model’s performance, especially in answering consumer medical questions. To address these problems, researchers introduced a method known as on-the-fly instruction tuning. This technique efficiently aligns LLMs to new domains using a small number of models, creating Med-PaLM. The Med-PaLM model, although it performs encouragingly and shows improvement in comprehension, knowledge retrieval, and reasoning, still falls short compared to clinicians.
One notable aspect of this research is the detailed human evaluation framework. This framework evaluates model answers for agreement with scientific consensus and potential harmful outcomes. For example, while only 61.9% of long Flan-PaLM answers are in line with scientific consensus, this number rose to 92.6% for Med-PaLM, compared to answers provided by clinicians. Likewise, the likelihood of adverse outcomes was significantly reduced in Med-PaLM responses compared to Flan-PaLM.
Human evaluation of Med-PaLM responses highlighted its efficiency in several areas, closely aligning with the answers provided by clinicians. This underscores the potential of Med-PaLM as a supportive tool in clinical settings.
The research discussed above delves into the complexities of enhancing large language models (LLMs) for medical applications. The techniques and observations from this study can be generalized to improve LLM capabilities across various fields. Let’s explore these key aspects:
Tuning instructions improves performance
- Generalized application: Instruction tuning, which involves tuning the LLM with specific instructions or guidelines, significantly improved performance across domains. This technique can be applied to other fields such as legal, financial, or educational fields to enhance the accuracy and relevance of LLM outputs.
Scale the model size
- Wider implicationsNote that changing the model size leads to improved performance is not limited to answering medical questions. Larger models, with a greater number of parameters, have the ability to process and generate more accurate and complex responses. This expansion can be useful in areas such as customer service, creative writing, and technical support, where accurate understanding and response generation is crucial.
Chain of Thought (COT) claim
- Use various fields: Using COT prompting, although it does not always improve performance in medical datasets, can be valuable in other fields that require complex problem solving. For example, in technical troubleshooting or complex decision-making scenarios, a COT prompt can direct LLMs to process information step-by-step, resulting in more accurate and rational outputs.
Self-consistency to enhance accuracy
- Wider applications: The self-consistency technique, where multiple outputs are generated and the most consistent answer is chosen, can significantly enhance performance in various domains. In areas such as finance or legal where accuracy is crucial, this method can be used to verify the generated outputs for higher reliability.
Uncertainty and selective forecasting
- Relevance across domains: Communicating uncertainty estimates is critical in fields where misinformation can have serious consequences, such as health care and law. Using LLMs’ ability to express uncertainty and selectively defer predictions when confidence is low could be a critical tool in these areas to prevent the dissemination of inaccurate information.
The real-world application of these models extends beyond answering questions. They can be used to teach patients, aid in diagnostics, and even train medical students. However, their deployment must be carefully managed to avoid relying on AI without proper human oversight.
As medical knowledge evolves, LLM holders must also adapt and learn. This requires mechanisms for continuous learning and updating, ensuring that models remain relevant and accurate over time.